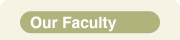
- Professor
- Fellow Professor
- Associate Professor
- Lecturer
- Assistant Professor
Japanese

|
Name |
Masaharu Adachi |
Position |
Professor |
Degree(s) |
Ph.D. |
Main Subjects |
Fundamentals of Electrical Circuit Electric Circuit Theory and Practice I System Engineering Engineering English Workshop |
Specialty |
Nonlinear system engineering |
Field of Research |
Supervised and unsupervised learning for artificial neural networks. Associative memory especially with chaotic dynamics. Nonlinear time series prediction. |
Academic Society |
IEEE |
Short Curriculum Vitae |
Mar. 1989 : B.E. degree in electronic engineering from Tokyo Denki University
Mar. 1994 : Ph.D. from Tokyo Denki University
Apr. 1994 : Research Officer of The University of Western Australia
May. 1995 : Postdoctral Researcher of RIKEN Institute
Oct. 1998 : Assistant Professor
Oct. 2000 : Associate Professor
Oct. 2006 : Professor
|
Mail |
 |
Selected Papers
- Yusuke Tabata and Masaharu Adachi: A Spiking Network of Hippocampal Model Including Neurogenesis, Lecture Notes in Computer Science, vol. 5506/2009, pp.14–21, 2009.
- Norihisa Sato and Masaharu Adachi: Synchronization of Chaotic Systems without Direct Connections Using Reinforcement Learning, IEICE Transactions on Fundamentals of Electronics, Communications and Computer Sciences, vol.E92-A, no.4, pp.958–965, 2009.
- Masaharu Adachi and Kazuyuki Aihara: An Analysis on Instantaneous Stability of an Associative Chaotic Neural Network, International Journal of Bifurcations and Chaos, vol. 9, no. 11, pp. 2157–2163, 1999.
- Masaharu Adachi and Kazuyuki Aihara: Associative Dynamics in A Chaotic Neural Network, INNS Neural Networks, vol. 10, no. 1, pp. 83–98, 1997.
- Masaharu Adachi and Makoto Kotani: Identification of Chaotic Dynamical Systems with Back-Propagation Neural Networks, IEICE Transactions on Fundamentals of Electronics, Communications and Computer Sciences, vol. E77-A, pp. 324–334, 1994.
Laboratory Introduction
The laboratory members work for the following projects.
- Improveing learning rules both of supervised and unspervised learning.
- Associative memory especially with chaotic dynamics.
- Application of artificial neural networks especially on time series prediction and analysis of functional near-infrared spectroscopy data.
Learning Systems Laboratory
Back to Top